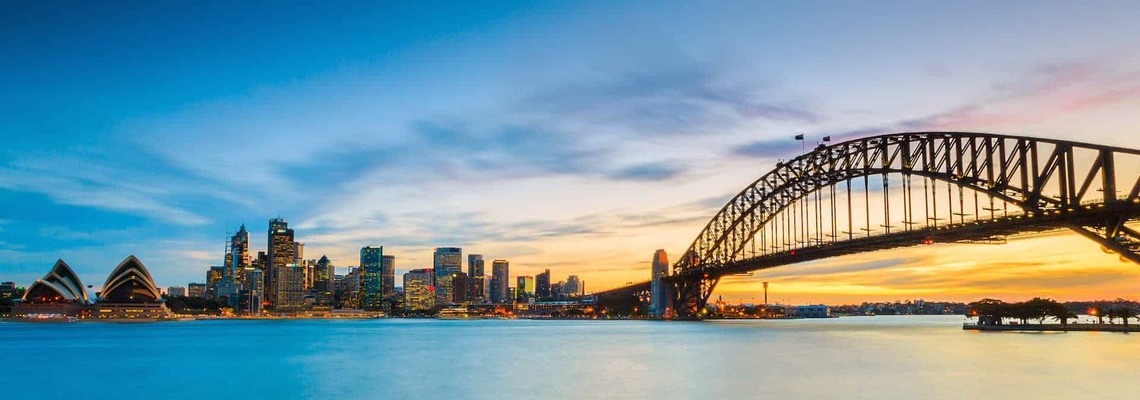
Speakers
Keynotes
Prof Lei Chen , Hong Kong University of Science and Technology
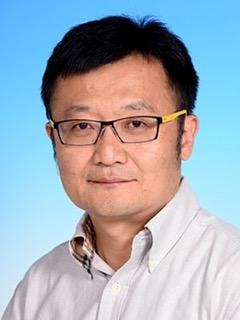
Bio: Lei Chen has BS degree in computer science and engineering from Tianjin University, Tianjin, China, MA degree from Asian Institute of Technology, Bangkok, Thailand, and PhD in computer science from the University of Waterloo, Canada. He is a chair professor in the Department of Computer Science and Engineering, Hong Kong University of Science and Technology (HKUST). Currently, Prof. Chen serves as the head of Data Science and Analytic trust at HKUST (GZ), director of Big Data Institute at HKUST, director of HKUST MOE/MSRA Information Technology Key Laboratory. Prof. Chen’s research interests include human-powered machine learning, crowdsourcing, Blockchain, graph data analysis, probabilistic and uncertain databases and time series and multimedia databases. Prof. Chen got the SIGMOD Test-of-Time Award in 2015.The system developed by Prof. Chen’s team won the excellent demonstration award in VLDB 2014. Prof. Chen has served as VLDB 2019 PC Co-chair and Editor-in-Chief of VLDB Journal. Currently, Prof. Chen serves as Editor-in-Chief of IEEE Transaction on Data and Knowledge Engineering and PC Co-chairs of IEEE Conference on Data Engineering (ICDE 2023). He is an IEEE Fellow, ACM Distinguished Member and an executive member of the VLDB endowment.
Title: Data Management for Effective and Efficient Deep Learning
Abstract: In recent years, deep learning (DL) has significantly penetrated and has been widely adopted in various fields of application, including facial recognition, strategy games (AlphaGo and Texas hold'em) and question answering. However, the effectiveness of the models and efficiency of the training process strongly depend on how well the associated data is managed. It is very challenging to train an effective deep learning-based image classifier without properly labelled training data. Furthermore, training efficiency is severely affected by a large amount of training data, complex structures of the models and tones of hyper parameters. A lack of validation for result data and explanation also seriously affect the applicability of trained models. In this talk, I will discuss three issues on how to manage data for effective and efficient deep learning: 1) how to prepare data for effective DL, which includes data extraction and integration as well as data labelling; 2) how to reduce the training time with data compression, computation graph optimization and tensor program reuse, and 3) how to conduct explanation to make the model robust and transparent. Some future work will be highlighted at the end.
Dr Divesh Srivastava, AT&T
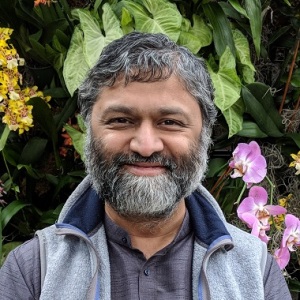
Bio: Divesh Srivastava is the Head of Database Research at AT&T. He is a Fellow of the ACM, the President of the VLDB Endowment, co-chair of the ACM Publications Board, and on the Board of Directors of the Computing Research Association. He has served as PC co-chair of many international conferences including SIGMOD 2021, VLDB 2020 (Industrial), SIGMOD 2020 (Industrial), and ICDE 2019. He has presented keynote talks at several international conferences, and his research interests and publications span a variety of topics in data management. He received his Ph.D. from the University of Wisconsin, Madison, USA, and his Bachelor of Technology from the Indian Institute of Technology, Bombay, India.
Title: Exploring and Analyzing Change: The Janus Project
Abstract: Data change, all the time. The Janus project seeks to address the Variability dimension of Big Data by modeling, exploring, and analyzing such change, providing valuable insights into the evolving real world and the ways in which data about it are collected and used.
We start by identifying technical challenges that need to be addressed to realize the Janus vision. Towards this end, we have extracted and worked with the histories of various structured datasets, including DBLP, IMDB, open government data, and Wikipedia, for which a detailed history of every edit is available. Our DBChEx (Database Change Explorer) prototype enables interactive exploration of data and schema changes, and we show how DBChEx can help users gain valuable insights by exploring two real-world datasets, IMDB and Wikipedia infoboxes.
Based on an analysis of the history of 3.5M tables on the English Wikipedia for a total of 53.8M table versions, we then illustrate the rich history of structured Wikipedia data: we show that tables are created in certain locations, they change their shape, they move, they grow, they shrink, their data change, they vanish, and they re-appear; indeed, each table has a life of its own. Finally, to help automatically interpret the useful knowledge harbored in the history of Wikipedia tables, we present recent results on two technical problems: (i) identifying Natural Keys, a particularly important piece of metadata, which serves as a primary key in tables over time and consists of attributes inherent to an entity, and (ii) matching tables, infoboxes and lists within a Wikipedia page across page revisions. We solve these problems at scale and make the resulting curated datasets available to the community to facilitate future research.
This is joint work with Tobias Bleifuß, Leon Bornemann, Dmitri Kalashnikov, and Felix Naumann.
Tutorial
Prof Zhifeng Bao, RMIT Centre of Information Discovery and Data Analytics
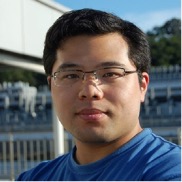
Bio: Prof Zhifeng Bao co-directs the RMIT Centre of Information Discovery and Data Analytics and leads the Big Data and Database Group in the Centre. He is also an Honorary Senior Fellow at The University of Melbourne. He obtained his PhD in Computer Science from National University of Singapore in 2011 and was the winner of the Best PhD Thesis Award. He received the Chris Wallace Award for Outstanding Research by the Computing Research and Education Association of Australasia (CORE) in 2020. He is a two-time winner of the Google Faculty Research Award. His research interest is big data management and mining, with a focus on relational data, geo-spatial data and graph data. Currently, his group is working on query optimization in database systems, data quality for ML, data/query pricing, and ML-enhanced algorithms.
Mr Hai Lan, RMIT Centre of Information Discovery and Data Analytics
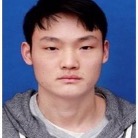
Bio: Mr Hai Lan is a current PhD candidate at RMIT University under the supervision of Prof Zhifeng Bao and Prof Shane Culpepper. He obtained his Bachelor’s degree and Master’s degree (supervisor A/Prof Yuwei Peng) in 2017 and 2020 respectively from Wuhan University. His research interests include query optimization, indexing techniques in the database system, ML for database problems, and trajectory processing and management.
Title: Learn to Index Your Data and Select Your Index
Abstract: Indexes are crucial components in database systems which enable more efficient data access. There are two fundamental problems in database indexing, (1) how to design an efficient index structure for a certain data type, and (2) how to select the suitable indexes to build to maximize the workload performance. With the rise of ‘ML for DB’, many research studies have explored how to solve the two problems above with the learned-based methods. Intuitively, in the former problem, the proposed methods learn the data distribution while in the second problem, the proposed methods find an optimal solution with the learned knowledge in a large solution space. Impressive results have been reported compared to the non-learning methods. In this tutorial, we discuss the design space of key methods for each problem above, present the details of the representative work, and provide some future research directions.
A/Prof Lu Qin , University of Technology Sydney
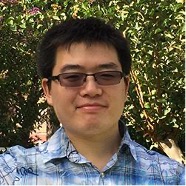
Bio: : Dr Lu Qin is an Associate Professor at the Australian Artificial Intelligence Institute (AAII), University of Technology Sydney (UTS). He is the director of the Large Network Analytics group (LNA) at AAII. His main research interest focuses on big graph analytics and processing, which has been a very hot research topic in the big data era. His dedication to research has resulted in two books and more than 140 conference/journal papers, all of which are ranked ERA A*/A. He received the Best Student Paper Award in ICDE 2007, the Best Paper Award in ICDE 2016, and the Best Paper Runner-up Award in VLDB 2021. He got ARC Discovery Early Career Researcher Award (DECRA) in 2014 and ARC Future Fellowship Award in 2020. He also got a leading CI ARC Discovery Project (DP) Funding in 2016 and another ARC Discovery Project Funding in 2021.
Title: Querying and Processing Bipartite Graphs: Models, Algorithms and Experiments
Abstract: Bipartite graph is a popular data structure, which has been widely used for modelling the relationship between two sets of entities in many real-world applications. For example, in E-Commerce, a bipartite graph can be used to model the purchasing relationship between customers and products. Due to the special structure of a bipartite graph, the algorithms to solve many fundamental problems in general graphs cannot be easily extended to handle bipartite graphs. For example, maximum clique computation is one of the most fundamental problems in general graphs. However, in bipartite graphs, the corresponding problem - maximum biclique computation - involves many new challenges, especially when handling large-scale graphs. In this talk, I will introduce the most recent studies on solving some fundamental problems in large-scale bipartite graphs, including butterfly counting, bi-clique search, core decomposition, bi-truss decomposition, bi-community search, and reachability processing. For each problem, I will first introduce the model and applications, and then explain the algorithms, and finally show the experimental results.
A/Prof Jianxin Li , Deakin University
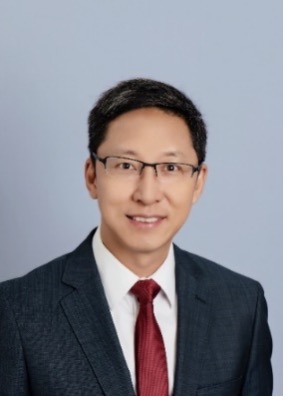
Bio: Dr Jianxin Li is an A/Professor of Data Science in the School of IT, Deakin University. His research interests include social computing, query processing and optimization, and big data analytics. He has published 130 high quality research papers in top international conferences and journals, including SIGMOD, PVLDB, ICDE, ACM WWW, SIGKDD, ACM CIKM, IEEE TKDE, TII, IS, etc. His professional service can be identified by different roles in academic committees, e.g., Editor-in-Chief in Array Journal, Associate Editors in Knowledge-based Systems, World Wide Web Journal, IEEE Signal Processing Letters, Information Systems; the PC co-chairs in DASFAA 2023, WISA 2022, BESC 2022, ADMA 2019; the General co-chairs in IEEE ISPA-2020, CIT-2021; guest editors and invited reviewers in many top international journals and technical program committee members in most world leading database and data mining international conferences like PVLDB, ICDE, WSDM, ICDM, AAAI, IJCAI.
Title: Temporal Community Mining, Computation and its Applications
Abstract: Searching for local and global communities is an important research problem that supports advanced data analysis in various complex networks, such as social networks, collaboration networks, cellular networks, etc. The evolution of such networks over time has motivated several recent studies to identify temporal communities in dynamic networks. At this tutorial, I will first provide a big picture to overview the diversified types of communities investigated in recent years. After that, I will introduce a set of representative works focusing on temporal community mining, efficient computational algorithms, and the emerging applications to be supported. Furthermore, I will share my team’s recent paper accepted by PVLDB 2022 - “reliable community search in dynamic networks”. In this work, we proposed a novel (, k)-core reliable community (CRC) model in the weighted dynamic networks, and defined the problem of most reliable community search that couples the desirable properties of connection strength, cohesive structure continuity, and the maximal member engagement. By taking this as an example, I will discuss some new types of temporal communities that should pay more attention in the near future. The main goal of this tutorial is to help audiences to know and understand the different applications of community discovery in need, how the community models are devised, the existing research challenges and the state-of-the-arts in this topic. This tutorial is suitable to broad audiences who have interest in database, social data analytics, data mining and AI-driven decision making.
Dr Renata Borovica-Gajic, University of Melbourne
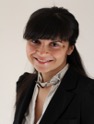
Bio: Dr Renata Borovica-Gajic holds a position of Senior Lecturer in Data Analytics in the School of Computing and Information Systems at The University of Melbourne. Dr Borovica-Gajic received her Ph.D. degree in Computer Science from Swiss Federal Institute of Technology in Lausanne (EPFL), Switzerland in 2016. Renata's research focuses on solving data management problems when storing, accessing and processing massive data sets, enabling faster, more predictable, and cheaper data analysis as a result. She envisions database systems as dynamic entities able to adjust query processing strategies to fit the characteristics of data and usage patterns. She is also interested in the topics of scientific data management, data exploration, query optimization, physical database design, and hardware-software co-design. Her work has repeatedly appeared in the premier data management conferences such as SIGMOD, VLDB, and ICDE, and has received a Test-of-Time award at SIGMOD 2022.
Title: Machine Learning and Databases: Friends or Foes?
Abstract: Machine Learning has revolutionized many domains, and is nowadays used in a wide range of applications such as internet ad placement, e-commerce rating systems, credit risk in finance, health analytics, and smart utility grids. In this talk we will consider whether databases are next in line. I will briefly cover the trends that led to a quick uptake of machine learning within the database engines, as well as discuss the current road blockers that prevent broader adoption within commercial database management systems. Finally, I will conclude the talk with a couple of examples of my work on using machine learning for database performance tuning and indexing, including recent ICDE 2021, ICDM 2021, and ADC 2020 publications.
Invited Talks
Mr Alexander Zhou , Hong Kong University of Science and Technology
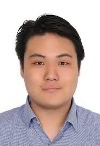
Bio: Mr Alexander Zhou is a current PhD Candidate at the Hong Kong University of Science and Technology under the supervision of Prof Lei Chen after completing his undergraduate studies at the University of Queensland. His research interests are on graph databases, taking a keen interest in communities and important subgraph structures on these networks. During his PhD studies, he has published twice as first author in the top database conference VLDB and collaborated with others on multiple other top conference publications.
Title: Beyond Normal Graphs: Finding New Research Topics on Complex Graph Types
Abstract: The traditional graph data representation is a vastly popular area of research with a plethora of ideas and discoveries. However, in the real world, graphs are often not simply 'normal' instead potentially having many unique properties or additional semantic information which hold the potential for even richer queries. Popular such settings of recent top-tier publications include Bipartite, Uncertain, Temporal, Distributed, HIN/Knowledge Graphs and many more, each with their own properties which can provide even more interesting queries and ideas. Using the well-established Clique structure as an example, we explore how by transporting it from normal graphs onto various alternative settings the structure changes and the information that the structure provides adapts in turn. By understanding each graph type and the potential queries it holds, exciting new research areas can open up.
Dr Jingwei Hou , University of Queensland
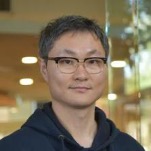
Bio: Dr Jingwei Hou obtained his PhD in Chemical Engineering from UNSW in 2015, and then he continued his postdoc research at the UNESCO Centre for Membrane Science and Technology (2015-2017) and the University of Cambridge (affiliate of the Trinity College, 2017-2019). He joined the University of Queensland in 2019 as an ARC DECRA Fellow and then named as the ARC Future Fellow in 2021. He is now a Senior Lecturer (continuing) and group leader at the University of Queensland.
Title: Three Lessons I Learnt During my PhD
Abstract: Doing research has never been easy - basically we are working at the boundary of science, doing things that have never been done before. What is more difficult is how to survive in the academic world as a researcher. You have to be really multi-tasking, capable of delivery high impact research, good teaching, service and of course constantly bring-in research grants to support yourself and the team. I would like to share some of my journeys along the way, and hopefully this can shed some lights on young PhD students and ECRs for pursuing their career path.